Understanding the Significance of Bounding Box in Data Annotation
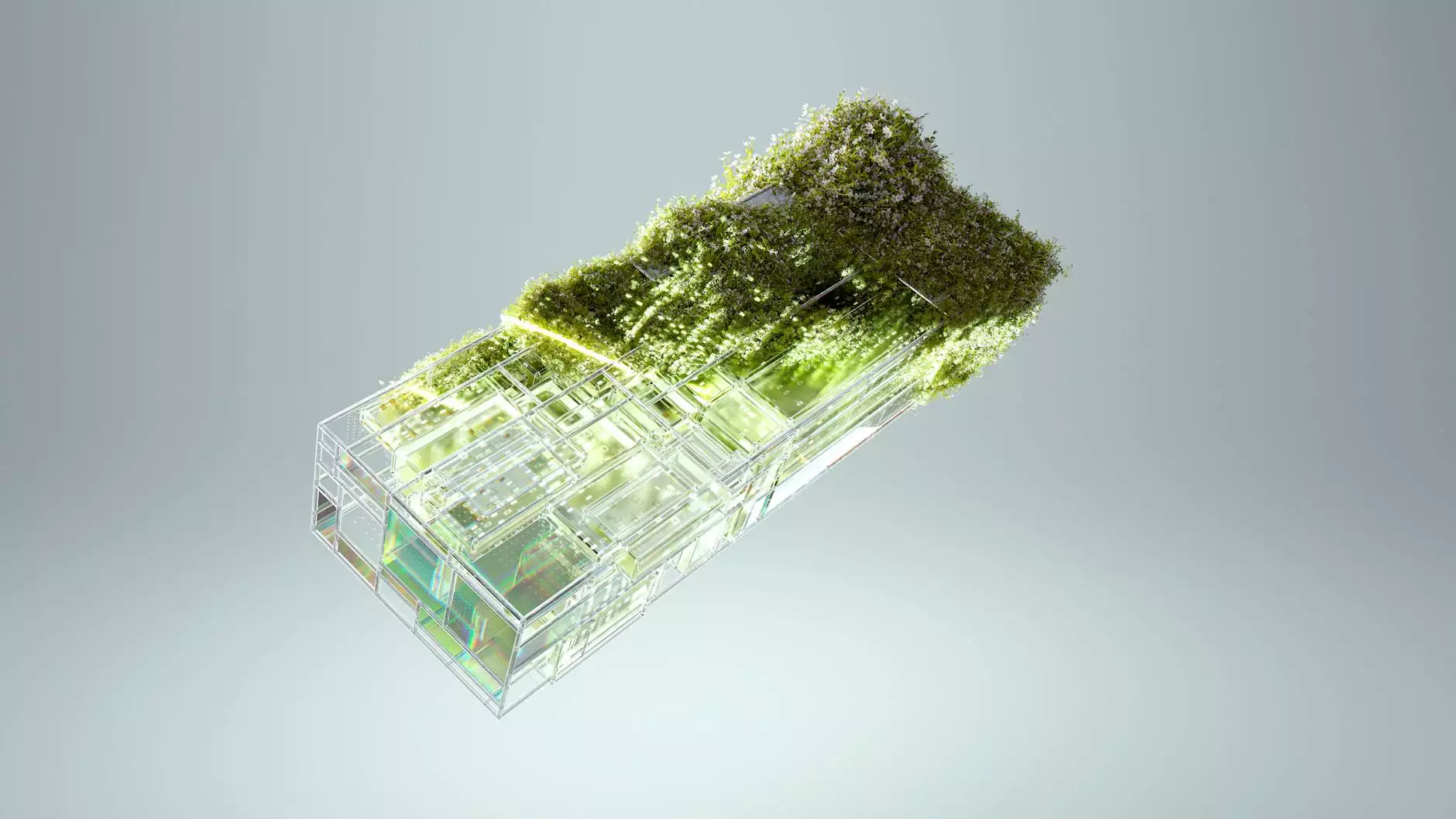
In the rapidly evolving field of artificial intelligence (AI) and machine learning (ML), the term bounding box has become a cornerstone concept within the realm of data annotation. As businesses and research institutions increasingly rely on annotated datasets to train their algorithms, understanding what bounding boxes are and how they are utilized can substantially affect the quality and effectiveness of AI models.
What is a Bounding Box?
A bounding box is defined as a rectangle that surrounds or encapsulates an object or a set of points in a two-dimensional or three-dimensional space. In the context of image processing and computer vision, a bounding box is a crucial tool used to identify objects within images, providing a geometric representation encapsulating the boundaries of the object of interest.
Why Are Bounding Boxes Important?
- Object Detection: The primary use of bounding boxes lies in object detection, where the algorithm learns to understand and identify objects in a scene, such as cars, pedestrians, traffic signs, and more.
- Data Annotation: Bounding boxes are integral to the data annotation process, enabling the labeling of images for training machine learning models. Accurate annotation is critical for achieving high performance in AI systems.
- Model Training: A well-defined bounding box allows algorithms to better understand and classify images accurately, improving their predictive capabilities.
- Evaluation Metrics: Bounding boxes facilitate the evaluation of detection algorithms by offering metrics such as Intersection over Union (IoU), which assesses how well the predicted output aligns with the ground truth.
Applications of Bounding Boxes in Various Domains
Bounding boxes are not limited to just one field; their applications span various industries, driven by the need for visual recognition and automated decision-making systems.
1. Autonomous Vehicles
In the automobile industry, particularly in the development of self-driving cars, bounding boxes play a pivotal role in detecting other vehicles, pedestrians, and obstacles in real-time. By providing spatial coordinates of surrounding objects, autonomous systems can make informed driving decisions.
2. Retail and Inventory Management
Retailers utilize bounding boxes for effective inventory management and customer behavior analysis. By analyzing customer interactions with products, businesses can optimize their layouts and stock levels based on visual object recognition.
3. Security and Surveillance
In security applications, bounding boxes are used to monitor and identify suspicious activities through video analytics. Automated systems process live feeds, detecting anomalies in behavior, and sending alerts, thus enhancing safety measures.
4. Medical Imaging
In the medical field, bounding boxes assist radiologists and medical professionals in identifying tumors and other critical anomalies in imaging scans. This capability improve diagnostic accuracy, allowing for early treatment interventions.
Creating Effective Bounding Boxes for Data Annotation
Building efficient bounding boxes is an essential skill for annotators in the data preparation phase for machine learning applications. The accuracy and precision of these bounding boxes directly impact the learning outcomes of AI models.
Best Practices for Bounding Box Annotation
- Be Precise: Ensure that the bounding box tightly fits around the object of interest. It should not include too much extra space around the object, as this may confuse the model during training.
- Label Consistently: Use consistent labeling conventions across similar objects. This coherence helps in standardizing the dataset, making it easier for models to learn.
- Multiple Objects: When multiple objects are present, each object should have its bounding box. Overlapping boxes should be avoided unless dealing with complex scenarios where occlusion occurs.
- Quality Control: Implement a rigorous quality control process to verify the accuracy of annotations. This may involve peer reviews or automated checks to ensure that the data is up to standard.
Benefits of Using a Data Annotation Platform with Bounding Box Capabilities
Utilizing a specialized data annotation platform such as KeyLabs.ai, provides numerous advantages in creating and managing bounding boxes effectively. Here are some notable benefits:
1. Enhanced Accuracy
Platforms that support bounding box annotation often come with tools and features that enhance accuracy, such as adjustable box dimensions, snap-to-object functionalities, and advanced editing capabilities.
2. Improved Efficiency
Modern annotation platforms offer automation and leveraging AI to streamline the labeling process, reducing the time and effort required. This efficiency ensures that large datasets can be processed quickly without sacrificing quality.
3. Collaboration and Scalability
With cloud-based solutions, teams can collaborate seamlessly on data annotation projects, ensuring that multiple annotators can work simultaneously. KeyLabs.ai offers scalable solutions to accommodate projects of any size.
4. Comprehensive Support
Choosing a robust data annotation platform provides users with comprehensive support. From onboarding assistance to detailed documentation, platforms like KeyLabs.ai ensure that users can maximize their annotation capabilities.
Challenges in Bounding Box Annotation
While the benefits of bounding box annotation are numerous, challenges do exist, and being aware of these can help in mitigating risks effectively.
1. Ambiguity in Object Boundaries
Defining the exact boundaries of objects, especially in complex scenes with occlusions or similar overlapping objects, can lead to ambiguous bounding boxes. This may require annotators to make subjective decisions that could affect outcomes.
2. Time Requirements
Careful annotation of bounding boxes is time-consuming, particularly when dealing with extensive datasets. Balancing speed and accuracy is often a critical challenge in processing large-scale annotations.
3. Data Quality Fluctuations
Variability in annotations can arise when multiple annotators work on different parts of the dataset. Consistent quality control measures must be established to ensure homogeneity in the dataset.
Future of Bounding Box Technology in AI
The future of bounding box technology within the field of AI looks promising, with advancements paving the way for improvements in various industries. As the demand for precise object detection grows, innovations in bounding box methodologies will likely continue to evolve.
1. Integration with 3D Technologies
The rise of 3D object recognition is leading to the development of 3D bounding boxes that represent volumes rather than areas. Such advancements will allow for more robust interactions in environments like virtual reality and augmented reality.
2. AI-Powered Tools
Tools that facilitate automatic bounding box generation using AI algorithms are revolutionizing the annotation process. These tools can significantly reduce human input, allowing annotators to focus on quality checks rather than initial box creation.
3. Real-Time Processing
The increasing processing power of machines suggests a shift towards real-time bounding box annotation, especially with the expanding areas of AR and live surveillance, where immediate responses are crucial.
Conclusion
In conclusion, the bounding box is more than just a simple rectangle; it is an indispensable component of data annotation that drives the success of various AI and ML applications. By understanding its significance and adhering to best practices, businesses and organizations can elevate their data quality, ultimately leading to superior AI outcomes.
Platforms like KeyLabs.ai are at the forefront of transforming how bounding boxes are utilized in data annotation, making them essential partners for businesses looking to enhance their machine learning capabilities. The journey of mastering bounding boxes is an exciting one, with vast potential for innovation and application in the future.